# Define a vector of numbers
<- c(1, 2, 3, 4, 5)
x
# Calculate the maximum
max(x)
## [1] 5
# function to repeat a variable multiple times
rep("test", 3)
## [1] "test" "test" "test"
# Concatenate strings, using "ing... " as the separator
paste(rep("test", 3), collapse = "ing... ")
## [1] "testing... testing... test"
10 Functions, Packages, and Environments
In addition to variables, functions are extremely important in programming. Functions allow you to repeat a series of steps using different information and get the result. In a way, a function is to a variable as a verb is to a noun - functions are a concise way of performing an action.
Packages contain groups of functions to accomplish tasks. In order to use functions from a package, you must install the package, and load it.
Environments are important for managing the set of installed packages that is available to use in a project. Environment management is different in R than it is in Python, and for a beginner, the R approach requires a bit less thought. For now, you will pick one Python environment management option and stick with it – later, you may develop opinions on which approach is useful for different tasks.
10.1 Objectives
- Use pre-written functions to perform operations
- Use python environments to manage packages
- Install and load packages in R and Python
- Use pipes to write readable code
- Functions are nicknames for blocks of code that execute a sequence of steps.
- Functions take arguments as parameters and return values
- Packages contain functions that are connected by a common goal or task sequence.
- Packages must be installed and loaded in order for the functions in the package to be used.
- Environments manage the available packages and functions that can be loaded and used.
10.2 Using Functions
Functions are sets of instructions that take arguments and return values. Strictly speaking, mathematical operators (like those above) are a special type of functions.
We’re not going to talk about how to create our own functions just yet. Instead, in this chapter, let’s figure out how to use functions.
It may be helpful at this point to print out the R reference card1 and the Python reference card.2 These cheat sheets contain useful functions for a variety of tasks in each language.
10.2.1 Function Vocabulary
Suppose I have a function called add(x, y)
which takes two numbers and adds them together.
In this example, add
is the function name, and x
and y
are parameters: placeholder names for information to be passed into the function. Not all functions have named parameters, but it is common for named parameters to provide some indication of what information is supposed to go in that spot.
When I call the function – that is, I use it to add two numbers together, I have to pass in arguments. Arguments are values which are assigned to parameters in the function and affect the result. This is pretty technical and a bit nit-picky, but it’s good to see information multiple times - we’ll revisit functions in Chapter 14.
The function call would be add(x = 3, y = 2)
, where 3 and 2 are the arguments. The function call would be evaluated and would return 5 as the answer (assuming that add
does what it says it does).
Let’s see these words in a more concrete setting.
which.max
and which.min
functionswhich.max
andwhich.min
are the function namesx
is the parameter
When I type which.max(x = c(2:10))
into the R console and hit Enter, - c(2:10) = c(2, 3, 4, 5, 6, 7, 8, 9, 10)
is the argument - inside which.max
, this argument has the name x
(mostly helpful for debugging) - which.max
will return 9, which is the index of x
with the largest value (10)
Methods are a special type of function that operate on a specific data type. In Python, methods are applied using the syntax variable.method_name()
. So, you can get the length of a string variable my_string
using my_string.length()
.
R has methods too, but they are invoked differently. In R, you would get the length of a string variable using length(my_string)
.
Right now, it is not really necessary to know too much more about functions than this: you can invoke a function by passing in arguments, and the function will do a task and return the value.
Try out some of the functions mentioned on the R and Python cheat sheets.
Can you figure out how to define a list or vector of numbers? If so, can you use a function to calculate the maximum value?
Can you find the R functions that will allow you to repeat a string variable multiple times or concatenate two strings?
Can you do this task in Python?
# Define a list of numbers
= [1, 2, 3, 4, 5]
x
# Calculate the maximum
max(x)
## 5
# Repeat a string multiple times
= ("test", )*3 # String multiplication
x # have to use a tuple () to get separate items
# Then use 'yyy'.join(x) to paste items of x together with yyy as separators
'ing... '.join(x)
## 'testing... testing... test'
10.2.2 Using R and Python as Overpowered Calculators
Now that you’re familiar with how to use functions, if not how to define them, you are capable of using R or python as a very fancy calculator. Obviously, both languages can do many more interesting things, which we’ll get to, but let’s see if we can make R and Python do some very basic stuff that hopefully isn’t too foreign to you.
Consider this triangle. I’ve measured the sides in an image editor and determined that \(a = 212\) pixels, \(b = 345\) pixels, and \(c = 406\) pixels. I suspect, however, that my measurements aren’t quite right - for one thing, I tried to measure in the center of the line, but it wasn’t as easy to do that well on the diagonal.
Let’s assume that my measurements for \(a\) and \(b\) are accurate and calculate how far off my estimate was for side \(c\). We will use the sqrt
function to accomplish this task – in R, sqrt()
, and in Python, math.sqrt()
. In Python we need to run import math
first to load the math library before we can use the math.sqrt
function.
# Define variables for the 3 sides of the triangle
<- 212
a <- 345
b <- 406
c_meas <- sqrt(a^2 + b^2)
c_actual
# Calculate difference between measured and actual
# relative to actual
# and make it a percentage
<- (c_meas - c_actual)/c_actual * 100
pct_error
pct_error## [1] 0.2640307
# To get the sqrt function, we have to import the math package
import math
# Define variables for the 3 sides of the triangle
= 212
a = 345
b = 406
c_meas = math.sqrt(a**2 + b**2)
c_actual
# Calculate difference between measured and actual
# relative to actual
# and make it a percentage
= (c_meas - c_actual)/c_actual * 100
pct_error
pct_error## 0.264030681414134
Interesting, I wasn’t as inaccurate as I thought!
Of course, if you remember trigonometry, we don’t have to work with right triangles. Let’s see if we can use trigonometric functions to do the same task with an oblique triangle.
Just in case you’ve forgotten your Trig, the Law of Cosines says that \[c^2 = a^2 + b^2 - 2 a b \cos(C),\] where \(C\) is the angle between sides \(a\) and \(b\).
I measure side \(a = 291\) pixels, side \(b = 414\) pixels, and the angle between \(a\) and \(b\) to be \(67.6^\circ\). What will I likely get for the length of side \(c\) in pixels?
Remember to check whether R and python compute trig functions using radians or degrees! As a reminder, \(\pi\) radians = \(180^\circ\).
# Define variables for the 3 sides of the triangle
<- 291
a <- 414
b <- 67.6
c_angle <- sqrt(a^2 + b^2 - 2*a*b*cos(c_angle/180*pi))
c_actual
c_actual## [1] 405.2886
I measured the length of side \(c\) as 407 pixels.
# To get the sqrt and cos functions, we have to import the math package
import math
# Define variables for the 3 sides of the triangle
= 291
a = 414
b = 67.6
c_angle = math.sqrt(a**2 + b**2 - 2*a*b*math.cos(c_angle/180*math.pi))
c_actual
c_actual## 405.28860699402117
I measured the length of side \(c\) as 407 pixels.
Congratulations, if you used a TI-84 in high school to do this sort of stuff, you’re now just about as proficient with R
and python
as you were with that!
10.3 Environments
You may have noticed in the Python example above, we had to import math
before we used the math.sqrt()
and math.cos()
functions. The math
package is a built-in package in Python, so we don’t have to install the package in order to use it (installing Python installs math
). But, before we can use math.sqrt()
, we have to import or load the math
package into our working space. In Python, this working space is called the object space; in R, it’s called the global environment. The terminology here differs between R and Python (which is confusing), but conceptually, it’s important to distinguish between the set of things that are available to use when you are writing a program and the set of things that are available to load.
Imagine that you’re an accomplished programmer, and you are juggling multiple different projects. Each project uses some of the same packages, but some different packages as well. You open up a project that you haven’t run in a year, and you find out that one of the packages you’ve updated more recently breaks a bunch of code you wrote a year ago, because the functions in the package have been renamed.
What could prevent this from happening?
One way to solve this problem is to store the packages used in each project inside the project directory, in what we might call a project environment or virtual environment. This will keep each project isolated from the others, so that if you update a package in one project, it doesn’t affect any other project.
However, this approach results in a lot of duplication: for one thing, you have copies of each package hanging around in every folder on your computer. That’s not storage efficient, but it does keep your code from breaking as frequently.
Python programmers prefer the project-specific approach, while R programmers default to installing packages at the user or system level.
10.3.1 Vocabulary by Analogy: Functions, Packages, Environments, and Repositories
Think of a package as a book, with each page of the book containing a specific function. The package repository (CRAN, PyPi, etc.) is a set of packages, roughly corresponding to a physical library or a bookstore - you can access the packages and install them (take them home). Unlike a physical library, though, usually you don’t have to return the packages you’ve checked out! The set of packages you have installed corresponds to the books you have at home. You can use any of the functions (pages of those books) when you want to program (or access information).
Image Source
How the packages are organized can reasonably differ based on how you prefer your house to be arranged, just as package organization differs significantly in R and Python.
I have a collection of books in multiple rooms of my house that are sorted by task and audience – the programming books are by my desk, the fiction books are near the couch, and the childrens’ books are in my kids’ bedroom. This roughly corresponds to Python-style virtual environments - the packages I need for each project are in the project location, rather than stored centrally.
Some people, on the other hand, prefer to keep all of the books that they’re not actively using on a centrally located set of bookshelves. This would correspond more closely to R package management - packages are installed in one place for the whole system to use.
Regardless of how packages are managed (virtual environments or centrally), to access the package’s functions, I have to go get the book and open it. This step corresponds most closely to loading a package into your global environment/object environment. The global environment (R) or object space (Python) is the collection of objects (functions, variables, etc.) that are immediately available to the user.
Again, R and Python prefer to manage this step differently. In R, functions from all loaded packages are available to the user directly using the function name. When multiple packages with lots of functions are loaded, this can be … messy, as in Figure 10.2 (a). In Python, the recommended way to load packages is to import the package and possibly give it a shorter alias; the functions must still be referenced as pkg.functionName
or alias.functionName
instead of just functionName
. This more closely matches Figure 10.2 (b), where pages are still contained in notebooks, and thus require an extra step to access.
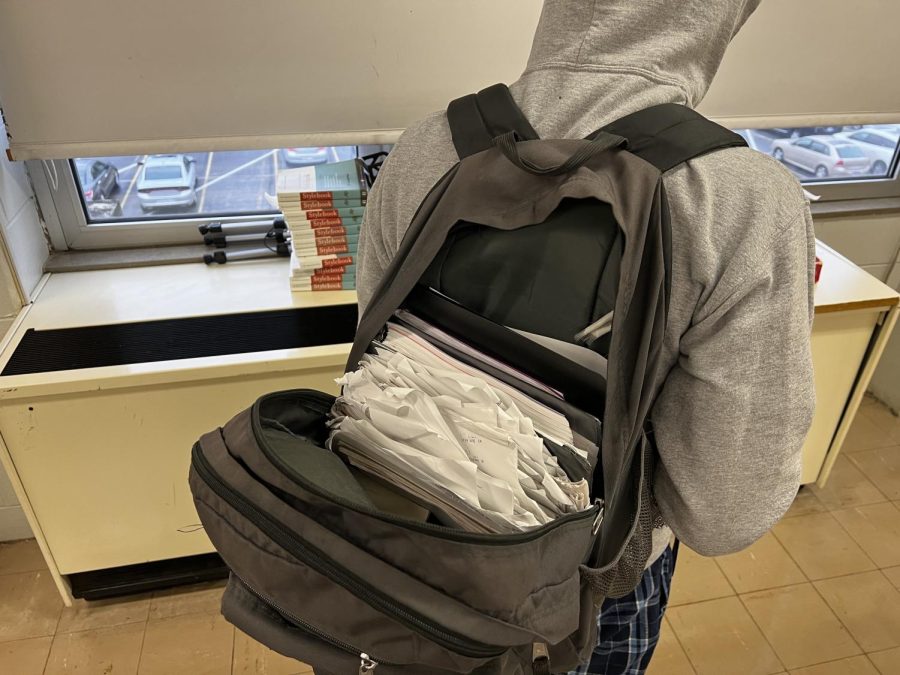
Image source
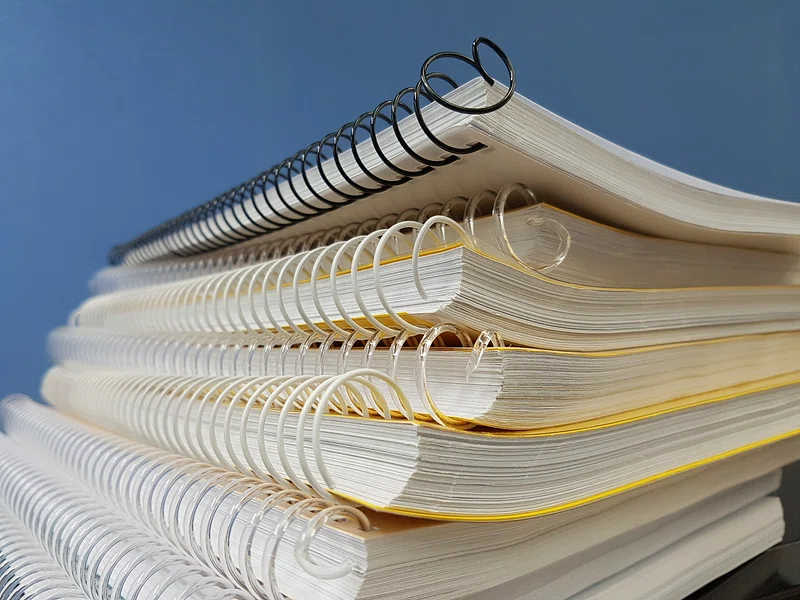
Image source
Some R programmers have adopted the python philosophy of project-specific package management, using an R package called renv
[1].
renv
documentation can be found here if you wish to try it out. I find that it is most useful for projects where package updates may break things - e.g. projects which run on shared systems or which are intended to work for a long period of time without maintenance.
If you want to use renv
, you can do that by following these steps:
install.packages("renv")
library(renv)
# Activate renv for a project
::activate()
renv
# this will install from github or CRAN
::install(c("pkg1", "pkg2", "githubuser/pkg3")) renv
I use renv
for this textbook, because if a package update breaks things, I need to systematically check all the code chunks in the textbook to make sure they all work. I don’t want to do that every time someone fixes a minor bug, so I don’t update the packages the textbook uses more than once a semester (normally).
10.3.2 Python Environments
In Python, packages are usually managed at the project level by creating virtual environments. The different environment management options in Python are one of the things that can make starting to learn python so difficult - it can be hard to make sure you’re using the right environment. virtualenv
and conda
are the main options for environment management. conda
is sometimes preferred for scientific computing because it handles the complex dependencies that arise from large packages like numpy
and scipi
and pandas
a bit better than pip
does alone.
By default, Chapter 2 just installs python at the system level.
If you don’t care about the nuances of which python environment management option you should use, follow the venv
instructions for Python below using the R console. venv
is relatively simple and straightforward and has less overhead.
I highly recommend that you pick one of these options and use that consistently, rather than trying the advantages and disadvantages of each option in different projects. If you switch around between virtualenv and conda, you can very quickly reach the point where you have 15 different python environments on your computer and you don’t have any idea which one you should be using. That is… not optimal, and one of the hardest things to deal with as a beginner Python programmer.
Python Environment, by Randall Munroe of [xkcd](https://xkcd.com/1987/). CC-By-NC-2.5.
The Python environmental protection agency wants to seal it in a cement chamber, with pictorial messages to future civilizations warning them about the danger of using sudo to install random Python packages.
10.3.2.1 venv
virtualenv
(venv
) can be installed using either RStudio or the system terminal.
Items within < >
(as well as the <> characters) are intended to be replaced with values specific to your situation.
10.3.2.2 Conda
conda
(aka Anaconda) can be installed using RStudio or the system terminal. You must have conda installed for these instructions to work. You can install conda system-wide by following these instructions, or, if you only intend to use Python within RStudio, you can install the reticulate
package and then run reticulate::install_miniconda()
to install miniconda to a directory where RStudio will be able to find it.
These steps have been generally constructed from [2].
Items within < >
(as well as the <> characters) are intended to be replaced with values specific to your situation.
10.4 Packages
Both R and python have a very robust system for extending the language with user-written packages. These packages will give you access to features that aren’t present in the base language, including new statistical methods, all sorts of plotting and visualization libraries, ways of interacting with data that are way more convenient than the default base language methods, and more.
10.4.1 Package repositories
Both R and Python have package systems, though generally, R is a bit more straightforward to deal with than python (in my opinion). Python’s extra environment management systems sometimes come with additional package repositories, and it can be hard to identify the differences between them. By contrast, all R packages seem to go through the same basic installation process and are just hosted in different places. This is largely a result of the difference between R and Python’s environment management strategies.
Formally Published | Informally Published/Beta | |
---|---|---|
R | CRAN, Bioconductor | github and other version control. See the remotes package documentation for all of the options. |
Python | PyPi | github and other version control systems |
There are tons of considerations to think about when using a new package, like how well it’s maintained, how many dependencies it has, and whether the developers of the package prioritize backwards-compatibility.
With each package you add, your project becomes more complex. On the other hand, with each package you add, you should be able to do more things, and hopefully, you’ll be able to leverage code from other developers to accomplish more complex tasks.
There’s a critical balance between complexity and trying not to reinvent the wheel. As you go through this book, you may want to consider the different packages presented in light of this complexity cost/benefit analysis.
Before we talk about how to install packages, we need to step back and think a little bit about the pros and cons of different ways of managing packages, because the most common R and python setups use very different approaches.
10.4.2 Package Installation
10.4.2.1 Installing packages in Python
Many of the instructions here are modified from [3].
Whichever method (venv, conda) you use to manage your Python environment, when you go to install a new package, you have a few different options for how to do so.
In python, you will typically want to install packages using a system terminal.
- Make sure your virtual environment/conda environment is activated
- Installation commands:
- If you are using venv,
pip3 install <package name>
should install your package. - If you are using conda,
conda install <package name>
is preferable, and if that doesn’t work, then try usingpip3 install <package name>
.
- If you are using venv,
# If you're using virtualenv
pip install <pkg1>
# If you're using conda, try this first
conda install <pkg1>
# If that fails, try pip
- Make sure R is using the correct python installation
- In the R terminal, run
reticulate::py_install("package name")
This is less elegant, but nearly foolproof because RStudio will install the package in the version of python it can find.
- At the top of the chunk, write
%pip install <package name>
- Run this code (Cmd/Ctrl + Enter)
- Comment the code out, so that you aren’t reinstalling the package every time you run the chunk.
%pip install <pkg1>
A slightly less elegant but more robust way to do this is to use the sys
package. Loading the sys
package ensures that you’re using the version of python that your file will be compiled with to install the package.
import sys
# For pip installation
!{sys.executable} -m pip install <pkg1>
# For conda installation
!{sys.executable} -m conda install <pkg1>
Once you’ve installed the package on your machine, you can comment these lines out so that they don’t run every time - this makes it a bit easier when you try to run old code on a new machine, as you can just uncomment those lines.
10.4.2.2 Installing packages in R
Package management in R is a bit simpler than package management in python.
In almost every case, you can install packages from CRAN with install.packages("package name")
. If your package is not on CRAN, and is instead on e.g. GitHub, you may have to use the remotes
package to install it with remotes::install_github("user/repo")
# CRAN packages
install.packages("<pkg1>")
# Github packages
::install_github("username/reponame") remotes
10.4.3 Loading Packages
Once you have the package installed, you need to load the package into memory so that you can use the functions and data contained within. Again, R and python differ slightly in how programmers conventionally handle this process.
- R: Load all of the package’s functions, overwriting already loaded functions if necessary
- Python: Load all of the package’s functions, contained within an object that is either the package name or a shortened alias.
Now, both R and python can load packages in either way, so this isn’t an either/or thing - it’s about knowing what the conventions of the language are, and then deciding whether or not it is appropriate to follow those conventions in your project. Figure 10.2 contains a visual analogy for the differences between these two approaches.
10.4.3.1 Import the whole package and all functions
To demonstrate this approach, let’s create a simple plot with a plotting library (ggplot2
in R, seaborn
in Python).
All of the other packages except for ggplot2
in this plot are present by default in any new R environment.
library(ggplot2)
<- search()
pkgs <- pkgs[grep("package:",pkgs)]
pkgs <- lapply(pkgs, function(x) as.character(lsf.str(x)))
all_fns <- data.frame(pkg = rep(pkgs, sapply(all_fns, length)),
pkg_fns fn = unlist(all_fns))
$pkg <- gsub("package:", "", pkg_fns$pkg)
pkg_fns
ggplot(pkg_fns, aes(x = pkg, y = after_stat(count), fill = pkg)) +
geom_bar() + theme(legend.position = "none") +
ylab("# Functions") + xlab("Package")
- 1
- List all containers that have been loaded (packages, but also things in the global environment and things that are autoloaded)
- 2
- Find only packages (discard things in the global environmente and autoloads)
- 3
- Get all functions available to the user in each loaded package
- 4
- Create a data frame with package and function names
- 5
- Remove “package:” from the package name
- 6
- Create the plot
I haven’t been able to figure out how to trace functions back to packages once they’re imported in Python, but we can create the same type of plot we did in R using the data frame of R functions in each R package. In python, if you want to import all functions from a package and use them with bare function names, you’d use from <pkgname> import *
.
from seaborn import *
import matplotlib.pyplot as plt
= countplot(r.pkg_fns, x = "pkg", hue = "pkg")
plot "Number of Functions in Common R Packages")
plot.set_title("Package")
plot.set_xlabel("# Functions")
plot.set_ylabel( plt.show()
In python, there are built-in functions (builtins
); I have then loaded the packages I typically use for plotting (seaborn
, seaborn.objects
, matplotlib
), manipulating data (pandas
, numpy
), and standard math
and statistics
libraries to roughly attempt to match the functionality available in base R + ggplot2.
import seaborn as sns
import seaborn.objects as so
import pandas as pd
import numpy as np
import matplotlib.pyplot as plt
import math
import statistics
= pd.DataFrame({"name": [
pkgs "builtins",
"math", "statistics",
"seaborn", "seaborn", "matplotlib",
"pandas", "numpy"
], "abbrev": [
"builtins",
"math", "statistics",
sns, so, plt,
pd, np]
})
"functions"] = pkgs['abbrev'].apply(lambda x: dir(x), by_row="compat")
pkgs[
= pkgs.explode('functions')
pkgs
= pkgs[~pkgs.functions.str.contains("__")]
pkgs
= sns.countplot(pkgs, x = "name", hue = "name")
plot "Number of Functions in Common Python Packages")
plot.set_title("Package")
plot.set_xlabel("# Functions")
plot.set_ylabel( plt.show()
- 1
- Create a data frame with all of the packages roughly corresponding to objects available in R after ggplot2 is loaded.
- 2
-
Find all functions in each package using
dir(x)
- 3
-
Expand the list of functions so that we have a simple data frame.
apply
created a list which was nested inside the data frame, so expanding it involves repeating the values in the un-nested rows for each item in the nested list. See Chapter 29 for more details of how this operation works. - 4
- Filter out functions that start with __ (these aren’t functions a user would typically call directly)
- 5
- Generate the plot
10.4.3.2 Use functions from the package without loading everything
# This code lists all the functions available to be called
<- search()
pkgs <- pkgs[grep("package:",pkgs)]
pkgs # get all the functions in each package that is loaded
<- lapply(pkgs, function(x) as.character(lsf.str(x)))
all_fns # create a data frame
<- data.frame(pkg = rep(pkgs, sapply(all_fns, length)),
pkg_fns fn = unlist(all_fns))
$pkg <- gsub("package:", "", pkg_fns$pkg)
pkg_fns
::ggplot(pkg_fns, ggplot2::aes(x = pkg, fill = pkg)) +
ggplot2::geom_bar(y = ggplot2::after_stat(count)) +
ggplot2::theme(legend.position = "none") +
ggplot2::xlab("Package") + ggplot2::ylab("# Functions")
ggplot2## Error: object 'count' not found
import plotnine as p9
= r.pkg_fns
pkg_fns
(= "pkg", fill = "pkg")) +
p9.ggplot(pkg_fns, p9.aes(x = p9.after_stat("count")) +
p9.geom_bar(y = "none") +
p9.theme(legend_position "Package") + p9.ylab("# Functions")
p9.xlab(
)## <plotnine.ggplot.ggplot object at 0x7f11d34a91d0>
In python, you can use import package as nickname
, or you can just use import package
and reference the package name directly. There are some packages which have typical aliases, and it’s best to use those so that you can look things up and not get too confused.
Package | Common Alias | Explanation |
---|---|---|
pandas | pd | shorter |
numpy | np | shorter |
seaborn | sns | This is a reference to Samuel Norman Seaborn, played by Rob Lowe, in the TV show The West Wing |
plotnine | p9 | |
BeautifulSoup (bs4) | bs | BeautifulSoup is a reference to Alice in Wonderland. The package name in PyPi is actually bs4. |
10.5 Pipes
Pipes are useful items for moving things from one place to another. In programming, and in particular, in data programming, pipes are operators that let us move data around. In R, we have two primary pipes that are similar (you may see both used if you google for code online). Any R version after 4.1 has a built-in pipe, |>
; the tidyverse
libraries use a pipe from the magrittr
package, %>%
.
For right now, it’s ok to think of the two pipes as essentially the same (but you can read about the differences [4]).
Fundamentally, a pipe allows you to take a function b()
and apply it to x
, like b(x)
, but write it as x |> b()
or x %>% b()
. This is particularly useful in cases where there are multiple sequential analysis steps, because where in regular notation you have to read the functions from the inside out to understand the sequential steps, with pipes, you have a clear step-by-step list of the order of operations.
In Python, there is a pipe
function in the Pandas library that works using .pipe(function)
notation [5]. From what I’ve seen reading code online, however, pipes are less commonly used in Python code than they are in R code. That’s ok - languages have different conventions, and it is usually best to adopt the convention of the language you’re working in so that your code can be read, run, and maintained by others more easily.
Generate 100 draws from a standard normal distribution and calculate the mean.
In R, simulate from a normal distribution with rnorm
. In python, use np.random.normal
- you’ll have to import numpy as np
first.
Use 3 approaches: 1. Store the data in a variable, then calculate the mean of the variable 2. Calculate the mean of the data by nesting the two functions (e.g. mean(generate_normal(100))
in pseudocode) 3. Calculate the mean of the data using the pipe (e.g. generate_normal(100) |> mean()
)
Consider: What are the advantages and disadvantages of each approach? Would your answer change if there were more steps/functions required to get to the right answer?
<- rnorm(100)
data mean(data)
## [1] -0.06622353
mean(rnorm(100))
## [1] 0.08116057
library(magrittr) # load the pipe %>%
rnorm(100) %>%
mean()
## [1] 0.01607093
rnorm(100) |> mean()
## [1] 0.0551973
In python, task 3 isn’t really possible, because of the way Python function chaining works, but task 2 is basically the equivalent.
import numpy as np
import pandas as pd
= pd.Series(np.random.normal(size = 100))
nums
nums.mean()## np.float64(0.0810200073777474)
=100).mean()
np.random.normal(size## np.float64(-0.10102203371548832)
The conclusion here is that it’s far easier to not use the pipe in python because the .function
notation that python uses mimics the step-by-step approach of pipes in R even without using the actual pipe function. When you use data frames instead of Series, you might start using the pipe, but only in some circumstances - with user-defined functions, instead of methods. Methods are functions that are attached to a data type (technically, a class) and only work if they are defined for that class - for instance, .mean()
is defined for both Pandas series and numpy arrays.